Highlights from Surfing Uncertainty by Andy Clark
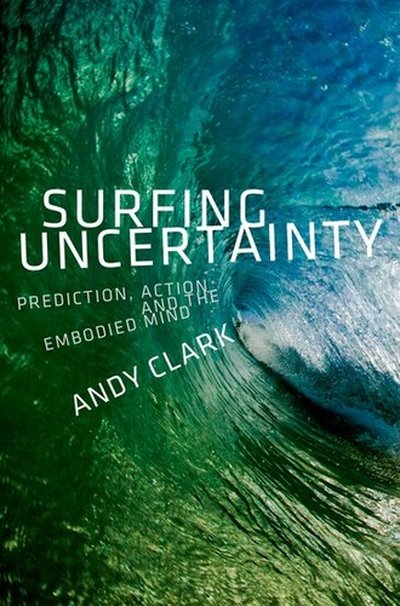
Highlights from this book
-
They deliver new accounts of the nature and structure of human experience.
-
perceiving itself involves a form of understanding:
-
By seeing brains as restless, pro-active organs constantly driven to predict and help bring about the play of sensory stimulation, we may be glimpsing some of the core functionality that allows three pounds or so of mobile, body-based brain-meat, immersed in the human social and environmental swirl, to know and engage its world.
-
perception is controlled hallucination.3 Our brains try to guess what is out there, and to the extent that that guessing accommodates the sensory barrage, we perceive the world.
-
Let’s take the training signal first. One way to think about prediction-driven learning is to see it as offering an innocent (that is, ecologically feasible) form of supervised learning. More accurately, it offers a form of self-supervised learning, in which the ‘correct’ response is repeatedly provided, in a kind of ongoing rolling fashion, by the environment itself.
-
predictive forms of learning are particularly compelling because they provide a ubiquitous source of learning signals: if you attempt to predict everything that happens next, then every single moment is a learning opportunity. This kind of pervasive learning can for example explain how an infant seems to magically acquire such a sophisticated understanding of the world,
-
The prediction task, thus conceived, is a kind of bootstrap heaven. For example, to predict the next word in a sentence, it helps to know about grammar (and lots more too). But one way to learn a surprising amount about grammar (and lots more too) is to look for the best ways to predict the next words in sentences. This is just the kind of training that the world can naturally provide, since your attempts at prediction are soon followed by the soundform corresponding to (you guessed it) the next word in the sentence. You can thus use the prediction task to bootstrap your way to the grammar, which you then use in the prediction task in future.
-
prediction-driven learning, as it unfolds in these kinds of multilayer settings, tends to separate out interacting distal (or bodily) causes operating at varying scales of space and time. This is important since structured domains are ubiquitous in both the natural and human-built world. Language exhibits densely nested compositional structure in which words form clauses that form whole sentences that are themselves understood by locating them in the context of even larger linguistic (and non-linguistic) settings. Every visual scene, such as a city street, a factory floor, or a tranquil lake, embeds multiple nested structures (e.g., shops, shop doorways, shoppers in the doorways; trees, branches, birds on the branches, leaves, patterns on the leaves). Musical pieces exhibit structures in which overarching sequences are built from recurring and recombinant sub-sequences, each of which has structure of its own. The world, we might reasonably suggest, is known by us humans (and doubtless most other animals too) as a meaningful arena populated by articulated and nested structures of elements.
-
‘between raw data seeking an explanation (bottom-up) and hypotheses seeking confirmation (topdown)’
-
Low-level visual input [is] replaced by the difference between the input and a prediction from higher-level structures … higher-level receptive fields … represent the predictions of the visual world while lower-level areas … signal the error between predictions and the actual visual input.
-
authors concluded, exactly in line (as they note) with predictive processing, that ‘our results support an account of perception as a process of probabilistic inference … wherein integration of top-down and bottom-up information takes place at every level of the cortical hierarchy’
-
An interesting upshot of this is that many visual illusions, as mentioned in 1.12, may nonetheless be best understood as ‘optimal percepts’. In other words, given the structure and statistics of the world we inhabit, the optimal estimate of the worldly state (the estimate that represents the best possible take on the incoming signal, given what the system already knows) will be the one that, on some occasions, gets things wrong. A few local failures, then, are just the price we pay for being able to get things right, most of the time, in a world cloaked by ambiguity and noise.
-
The largest contributor to ongoing neural response is the ceaseless anticipatory buzz of downwards-flowing neural prediction that drives perception and action in a circular causal flow. Incoming sensory information is just one further factor perturbing those restless pro-active seas. As ever-active prediction engines these kinds of brains are not, fundamentally, in the business of ‘processing inputs’ at all. Rather, they are in the business of predicting their inputs. This pro-active neural strategy keeps us poised for action and (as we shall later see) allows mobile, embodied agents to intervene on the world, bringing about the kinds of sensory flow that keep them viable and fulfilled.
-
We are not cognitive couch potatoes idly awaiting the next ‘input’, so much as proactive predictavores—nature’s own guessing machines forever trying to stay one step ahead by surfing the incoming waves of sensory stimulation.
-
The perceptual problems that confront us in daily life vary greatly in the demands they make upon us. For many tasks, it is best to deploy large amounts of prior knowledge, using that knowledge to drive complex proactive patterns of gaze fixation, while for others it may be better to sit back and let the world do as much of the driving as possible. Which strategy (more heavily input-driven or more heavily expectation-driven) is best is also hostage to a multitude of contextual effects. Driving along a very familiar road in heavy fog, it can sometimes be wise to let detailed top-down knowledge play a substantial role. Driving fast along an unfamiliar winding mountain road, we need to let sensory input take the lead
-
It copes, PP suggests, by continuously estimating and re-estimating its own sensory uncertainty. Within the PP framework, these estimations of sensory uncertainty modify the impact of sensory prediction error. This, in essence, is the predictive processing model of attention. Attention, thus construed, is a means of variably balancing the potent interactions between top-down and bottom-up influences by factoring in their so-called ‘precision’, where this is a measure of their estimated certainty or reliability (inverse variance, for the statistically savvy). This is achieved by altering the weighting (the gain or ‘volume’, to
-
The only hypothesis that can endure over successive saccades is the one that correctly predicts the salient features that are sampled. … This means that the hypothesis prescribes its own verification and can only survive if it is a correct representation of the world. If its salient features are not discovered,
-
Indeed, it seems very likely that for most creatures acts of deliberate imagining (which I suspect may require the use of self-cueing via language) are simply impossible. But creatures that are thus enabled to perceive a structured world possess the neural resources to generate, from the top-down, approximations to those same sensory states. There thus emerges a deep duality between online perception (as enabled by the predictive processing architecture) and capacities for the endogenous generation of quasi-sensory states.
-
In the absence of the driving sensory signal, there is no stable ongoing information (in the form of reliable, estimated-as-high-precision, prediction error) about low-level perceptual detail available to constrain the system, and hence no pressure to create or maintain a stable hypothesis at the lower levels of processing.
-
The bulk of our story so far has focused upon the use of stored knowledge to predict what might be thought of as a kind of ‘rolling present’. Obviously, these processes of prediction depend heavily upon past experiences. But that dependence does not (yet) involve the actual recollection of past experiences. Instead, the past there exists only as it is crystallized into the agent-inaccessible form of altered probability density distributions used to meet and to organize the incoming sensory flow.
-
The brain, they conclude, is ‘a fundamentally prospective organ that is designed to use information from the past and the present to generate predictions about the future’ (Schacter et al., 2007, p. 660). This may be the deep reason why episodic memory is fragile, patchy, and reconstructive since ‘a memory system that simply stored rote records would not be well-suited to simulating future events’ (Schacter and Addis, 2007a, p. 27; see also Schacter and Addis, 2007b). Schacter and Addis, like Suddendorf and Corballis, are especially interested in the relations between episodic memory and a certain form of ‘personal, episodic’ future thinking: one in which we mentally project ourselves ahead in time by simulating our own possible future experiences. I think we may now flag this as another important and distinctive manifestation of what already looks, from the PP perspective, to be a quite fundamental alignment between perception, recall, and imagination. Such alignment flows directly, or so I have been arguing, from the basic prediction-and-generative-model-based perspective on perception: a perspective that may thus offer an even broader framework within which to conceptualize the relations between recall (of various kinds) and imagination (of various kinds). More generally, what seems to be emerging is a view of memory as intimately bound up with constructive processes of neural prediction and
-
PP offers an attractive ‘cognitive package deal’ in which perception, understanding, dreaming, memory, and imagination may all emerge as variant expressions of the same underlying mechanistic ploy—the ploy that meets incoming sensory data with matching top-down prediction